How to Train AI on Company Data For Optimized Processes
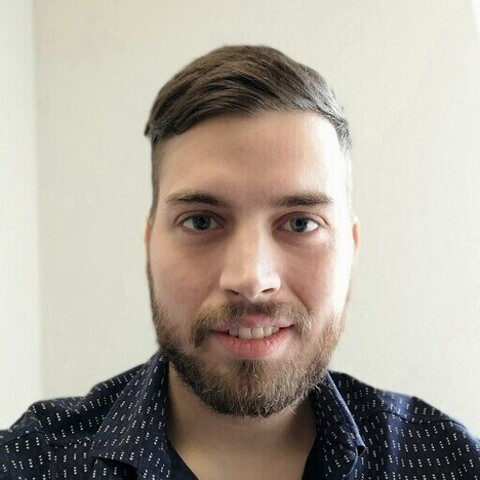
Companies generate daily data from emails, customer interactions, sales reports, website visits, and more. Using this data to train AI can create systems to understand and solve every business' unique challenges.
Instead of relying on one-size-fits-all AI models, you can train AI to understand your business language, answer customer questions, or search internal documents. And the best part? You don’t need to be a tech expert to get started.
In this guide, we’ll show you how to unlock the power of your existing data and turn it into AI solutions tailored to your specific needs.
We’ll also introduce you to Denser.ai, a user-friendly AI platform that will help you train AI models without needing any coding skills.
How to Train AI on Company Data Using Denser.ai
Training AI on your own company data can allow you to create a customized AI model that understands your specific business needs.
Whether you want a chatbot to improve customer support, automate internal document searches, or generate leads from your website, Denser.ai provides an easy way to integrate AI into your workflows.
Step 1: Sign Up for Denser.ai
Create a free account in minutes with Denser.ai and start training the chatbot using your company’s data.
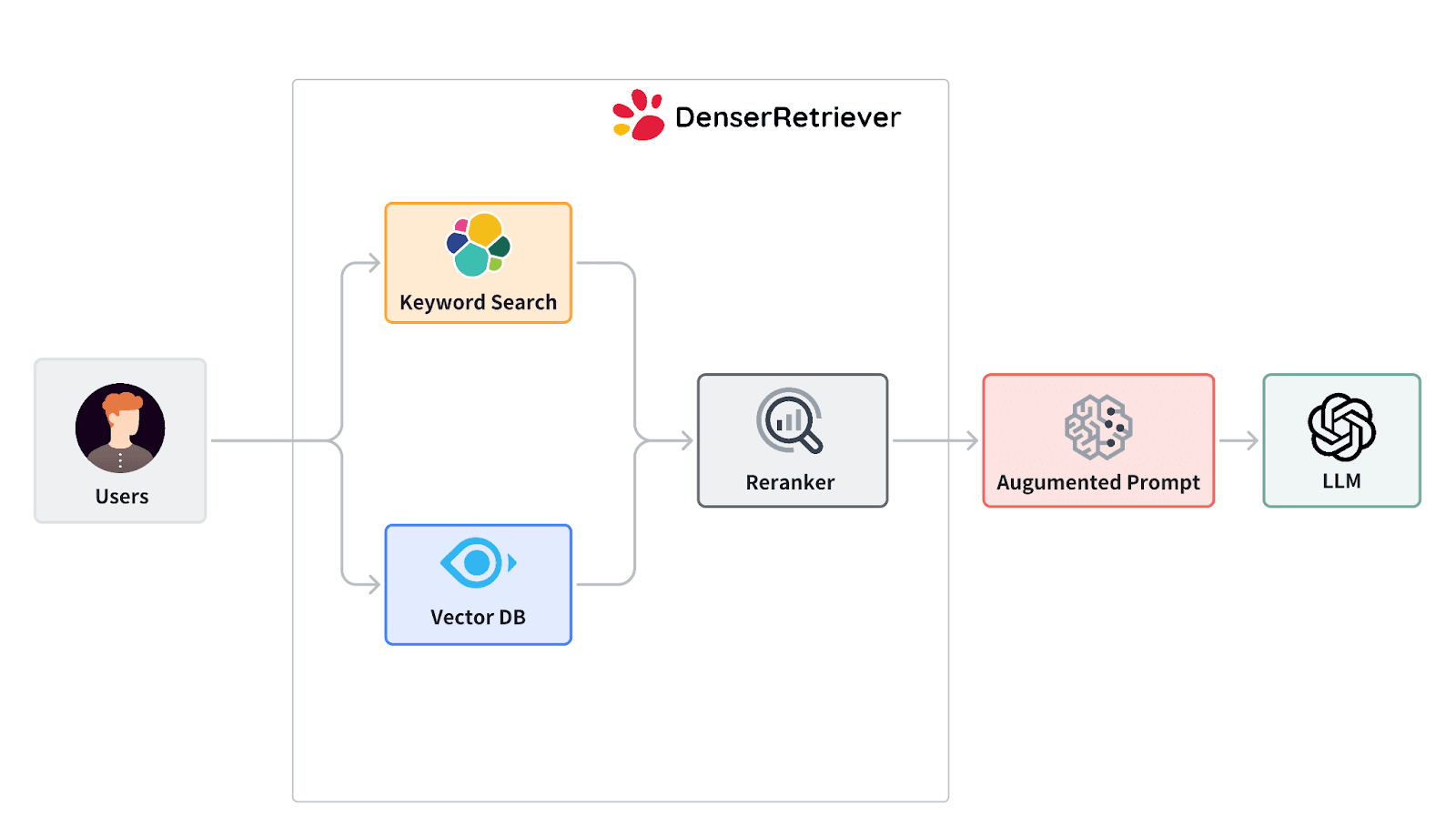
Denser.ai combines the best AI retrieval-based and generative AI models to make sure the AI delivers accurate and relevant content. This powerful generative AI tool is designed to find information quickly while maintaining exceptional accuracy.
The platform’s Denser Retriever supports various search methods:
- Keyword search: Ideal for simple, direct queries
- Vector search: Uses AI to find related information even when keywords aren’t an exact match
- Machine learning models reranking: Ensures the most relevant results are prioritized, delivering precise answers tailored to your data
With these capabilities, Denser.ai offers the best tool for businesses looking to incorporate and train generative AI using company data.
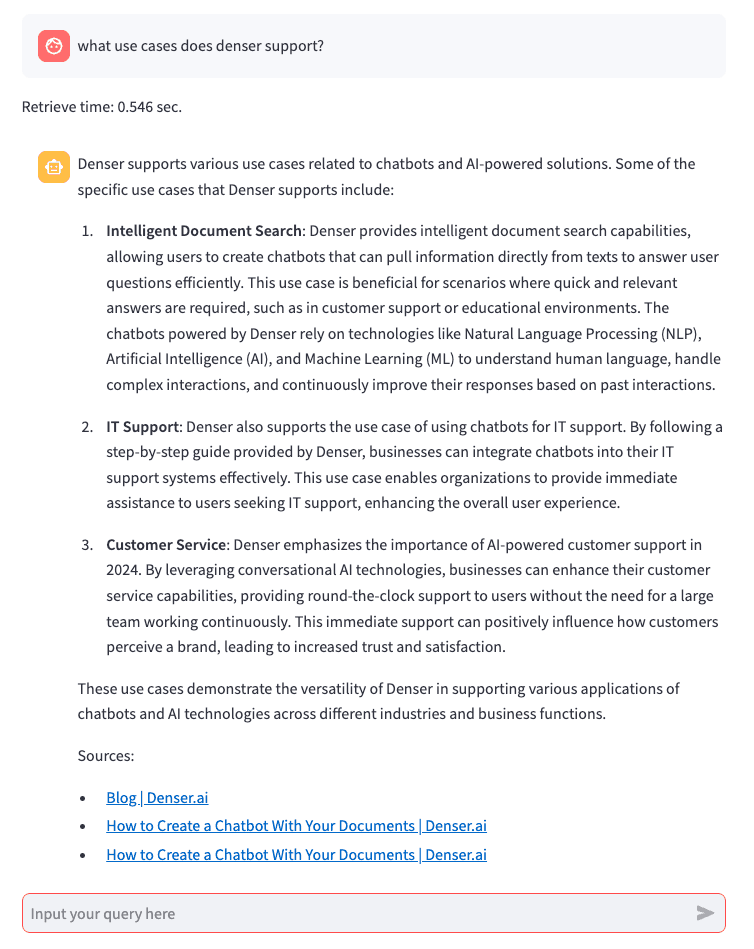
If you want a guided walkthrough, you can book a demo with a team member to understand how Denser.ai can meet your specific needs.
Try out a free trial or schedule a demo today!
Step 2: Create a New Chatbot
Once your account is set up, create a chatbot to provide accurate responses using your company’s data.
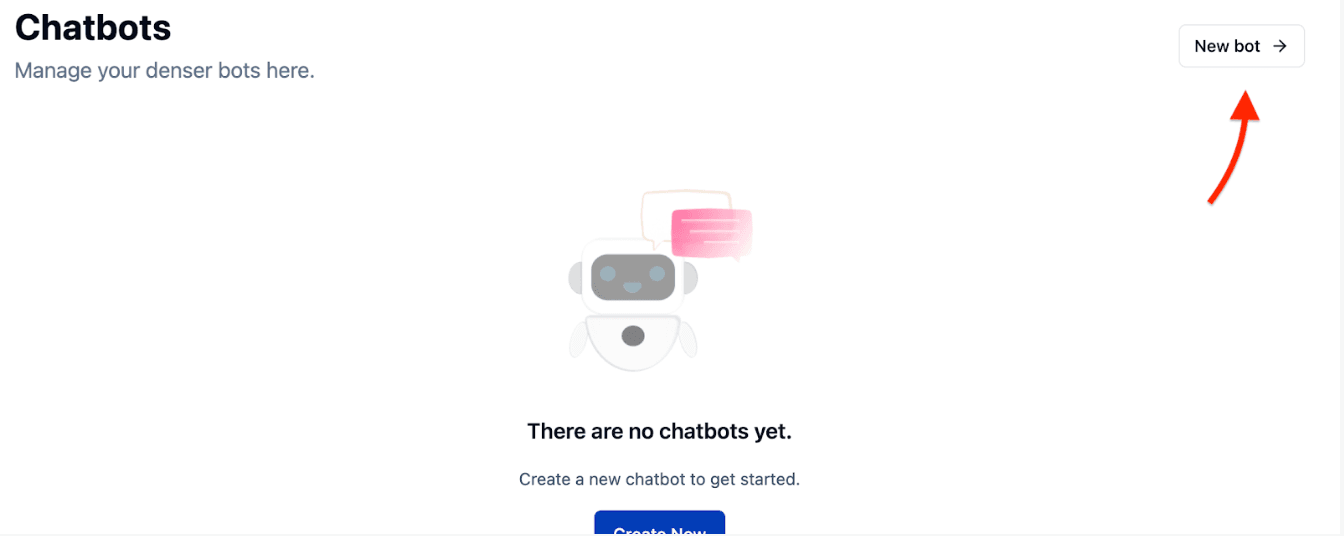
Click on the “Create New Chatbot” option within the Denser.ai dashboard. This is where you’ll configure the chatbot to learn from your data.
You can name your chatbot and select the type of data it will use (e.g., customer support data, internal documents, or product manuals).
Step 3: Upload Your Company Files
To train the AI, you’ll need to upload your documents, PDFs, spreadsheets, or other files containing valuable information your business relies on.
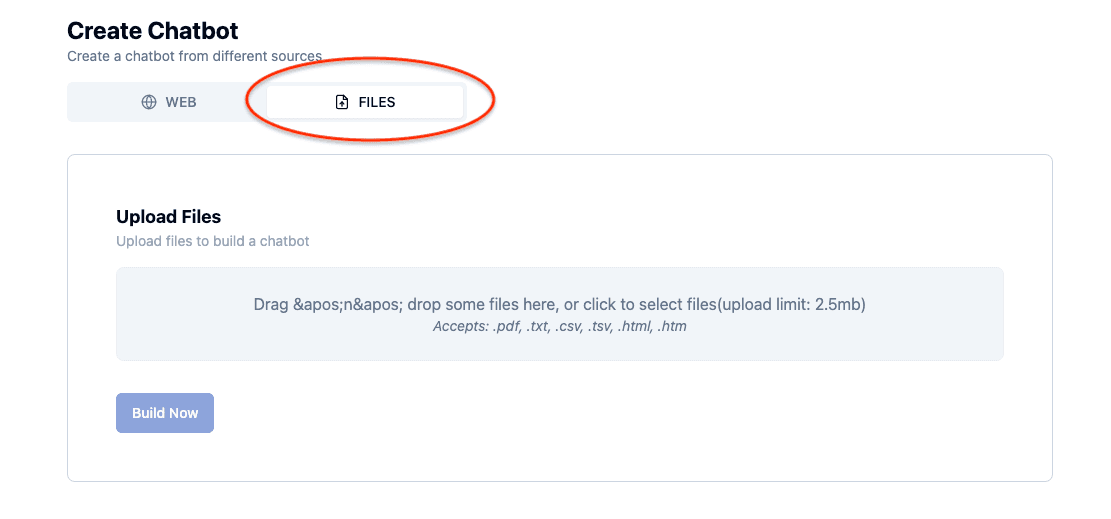
You can use various data types, such as:
- Customer support logs: For training chatbots to respond to common queries
- Sales records: To predict future sales trends or identify top-performing products
- Internal documents: Training AI to retrieve answers from company policies, manuals, or technical documentation
When using large language models, the quality of your data greatly impacts the accuracy of the AI’s responses.
You can drag and drop your files into the Denser.ai interface or use the file upload button. The platform will automatically process these files to prepare them for AI training.
Step 4: Train the AI Model Using Your Data
Once your files are uploaded, you can train the chatbot to understand and respond based on your specific company data.
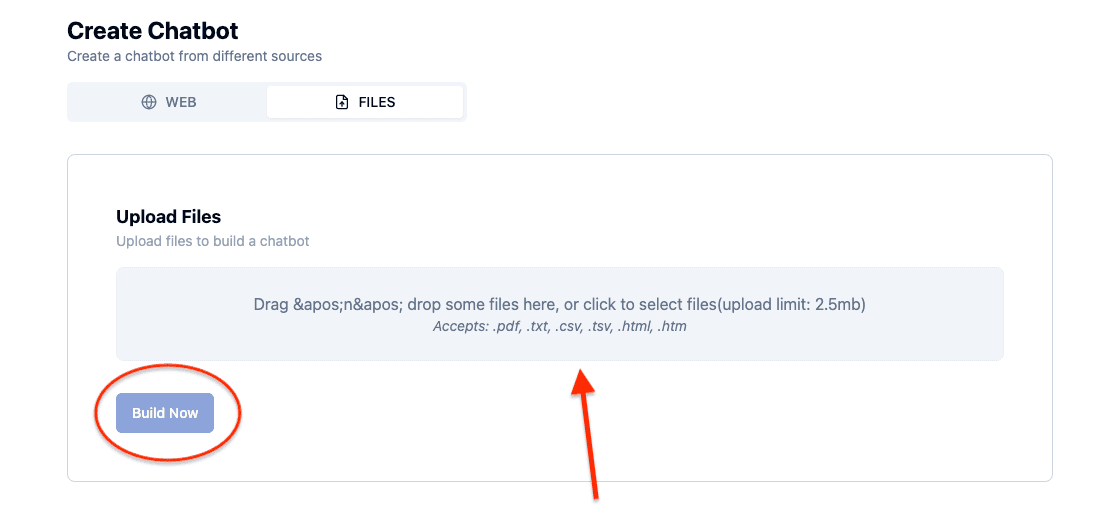
Click the “Build Now” button to start training the AI model. Denser.ai will use the information from your uploaded files to train the chatbot, turning your company’s data into actionable insights.
The more data you feed into Denser.ai, your chatbot becomes smarter and more accurate. It can learn from new inputs over time, adapting to changes in your business.
Step 5: Test and Deploy Your AI-powered chatbot
Now that your chatbot is trained on your data, it’s time to test it so it understands your content and provides accurate responses.
You can interact with the chatbot by asking questions related to the data you uploaded. This helps you see how well it understands and responds.
If the chatbot doesn’t answer correctly, you can refine its training by adding more data or adjusting the settings.
Once satisfied with the chatbot’s performance, deploy it on your website or integrate it with your internal systems to start automating tasks.
The Importance of Training AI on Company Data
Artificial Intelligence (AI) is becoming more common in businesses, but simply having it isn’t enough. The real value comes from training AI using your company’s own data.
Your data is unique to your business and contains insights that can help solve specific problems you face. Here’s why:
Tailored Solutions for Your Business Needs
When you train AI using your company’s data, it learns from the patterns and trends specific to your business. The AI becomes better at understanding your customers, products, and processes.
Examples:
If an online store uses AI trained on customer data, it can recommend products based on each customer's past purchases and browsing history. This makes the shopping experience more personal and relevant.
Training AI with your delivery data can help you predict which routes are most efficient if you run a logistics company. It can reduce fuel costs and improve delivery times.
Better Decision-Making
Training AI on your own data allows you to extract valuable insights that might go unnoticed. These insights can help you make better business decisions, which leads to growth and improved performance.
Analyzing customer interactions, such as emails, chat logs, and social media posts, can help the AI identify trends. It helps businesses adjust their marketing strategies to attract more customers.
Increased Automation and Efficiency
AI chatbots can be trained on past support tickets to automatically answer common questions. They can reduce the workload on your support team and speed up response times.
AI can also extract information from documents, such as invoices or contracts, and automatically enter it into your system.
Competitive Advantage
Using AI that is specifically trained on your data allows you to respond faster to market changes and customer needs. If your competitors use generic AI tools, their recommendations may not be as precise as yours.
Banks and financial institutions can train AI using their transaction data to detect unusual activity. It helps them catch fraud early, protecting the business and its customers.
Continuous Learning and Adaptation
AI models trained on your data can keep improving as new data comes in. The AI gets smarter over time, which makes predictions more accurate and efficient.
Businesses that sell seasonal products can update their deep learning models regularly to adjust to changing customer preferences. Therefore, marketing and inventory strategies are always in line with current trends.
Preparing Your Company Data for AI Training
The quality of your AI system depends heavily on the high-quality data you feed into it. Poorly prepared data can lead to inaccurate predictions, inefficient models, and wasted time and resources.
Preparing your data involves cleaning, organizing, and labeling it so the AI model can understand it and learn effectively. Let's go through the process of preparing your data for AI training.
Clean Your Data
Data cleaning involves various tasks, depending on the data type you are working with. Here are some of the most common tasks you will need to perform:
- Removing duplicates: If you have multiple entries of the same data, it can confuse the AI.
- Fixing errors: Check for incorrect entries, such as misspelled names or dates.
- Handling missing values: Fill in missing information or remove incomplete entries.
If your customer database has entries with missing phone numbers, you can either update the missing information or delete those entries if they are not critical.
Organize Your Data
If your data is well-structured, it will be easier to train, analyze, and maintain, which can save you time and resources in the long run.
To ensure consistency, you need to standardize the format of all your data. For example, if you are working with dates, ensure that all dates follow the same format (e.g., "YYYY-MM-DD"). Inconsistent formats can lead to errors during the training process.
Properly organizing your data into columns makes it easier for AI algorithms to understand. Think of each column as a specific feature from which the AI model can learn. If you have customer feedback, label it as “Complaint,” “Suggestion,” or “Compliment.”
Lastly, make sure the text data is consistent. For example, if customers use different terms for the same product (like “iPhone” vs. “i-phone”), standardize them.
Label Your Data for AI Training
Data labeling is tagging data with specific labels that help your AI model understand what each piece of information represents.
This is important for supervised learning, where the model needs labeled examples to learn how to make predictions.
If you’re training a chatbot, label previous customer inquiries by category, such as "Billing Issue," "Technical Support," or "General Inquiry." It helps the chatbot learn to respond accurately to similar questions in the future.
Manual labeling is precise but time-consuming. Consider using automated tools like Labelbox or Prodigy for large datasets to speed up the process.
Consistent labels are key—if you’re categorizing customer reviews, clearly define a “Positive” versus “Negative” review.
Train the AI Model
Once your data is cleaned, organized, and labeled, you can start training your AI model. This is the phase where the AI learns to recognize patterns based on the data you provide.
You can use Denser.ai to train a chatbot on customer support data to make it handle inquiries better. The training process involves feeding the data into the AI, allowing it to learn from the examples provided.
Challenges of Training AI on Company Data
While training AI using your company data can bring many benefits, it also comes with challenges. Addressing these challenges early on can save time, reduce costs, and help you get better results.
Data Privacy and Security Concerns
Privacy and security are major concerns when using internal data, especially data containing sensitive customer or financial information. Mishandling this data can lead to serious legal consequences, damage your reputation, and result in customer trust issues.
All customer data must be encrypted at rest (stored) and in transit (when sent across networks). You should also remove personally identifiable information (PII) to protect individual privacy.
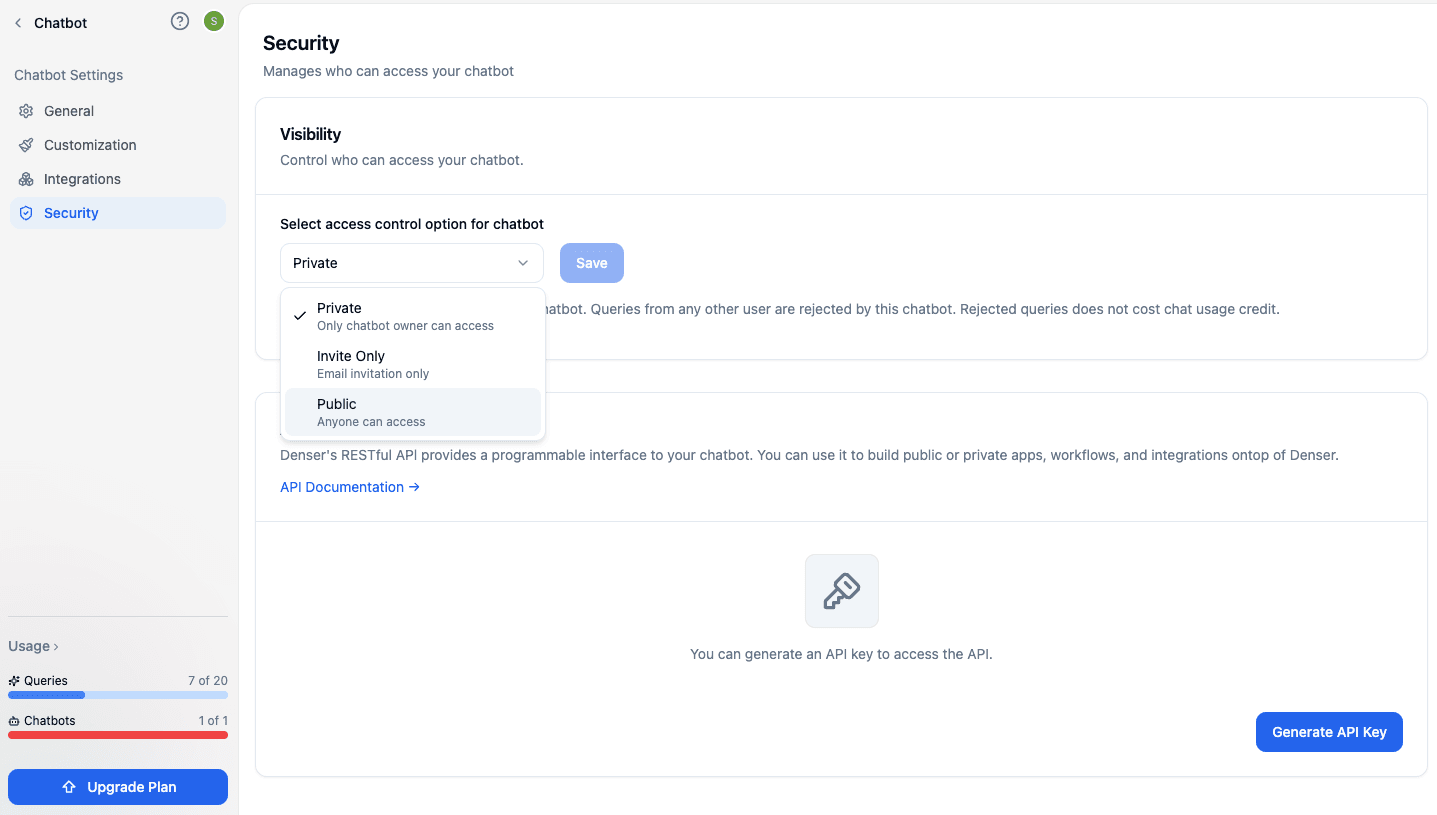
You must also limit who can access the data and the AI models. Only authorized team members should have access.
Potential Bias in Company Data
AI models are only as good as the data used to train them. If your data contains biases, the AI model will learn these biases, which may produce unfair or biased outcomes.
If your historical hiring data favors certain demographics, an AI model trained on that data could unintentionally discriminate in future hiring decisions.
Therefore, make sure to analyze past data to identify any patterns of bias. You must check if certain customer demographics are underrepresented. Include data from various sources to ensure the model has a broader understanding.
Data Integration from Multiple Sources
Many companies store their data in various formats and systems, such as spreadsheets, CRM systems, and email platforms. Integrating all this data into a single, consistent dataset can be challenging.
To resolve this, you must extract, transform, and load (ETL) tools like Apache Nifi or Talend to help combine data from multiple sources.
Also, store all your data in a centralized data warehouse like Amazon Redshift or Google BigQuery for easy access.
High Costs of Training AI Models
Training AI models, especially large ones, can be expensive in terms of time and resources.
Instead of setting up your own servers, you can use cloud services like AWS SageMaker or Google AI to pay only for what you use.
You may also use techniques like model pruning (removing unnecessary parts of the model) to reduce the computational load.
Pre-trained models can be fine-tuned with your data, which can save you time and money compared to building from scratch.
Your Data Holds the Key to Growth – Let Denser.ai Help
Are you looking to make better use of your company’s data? Denser.ai can help you train AI using your own data to solve your business challenges.
Instead of using one-size-fits-all AI models, Denser.ai lets you build tools that fit your business needs. It helps you build AI solutions tailored to tasks like customer support, document searches, or gaining insights from sales data—without needing any coding skills.
Denser.ai uses advanced search methods like keyword, vector, and machine learning model reranking to ensure your AI provides accurate, context-aware answers.
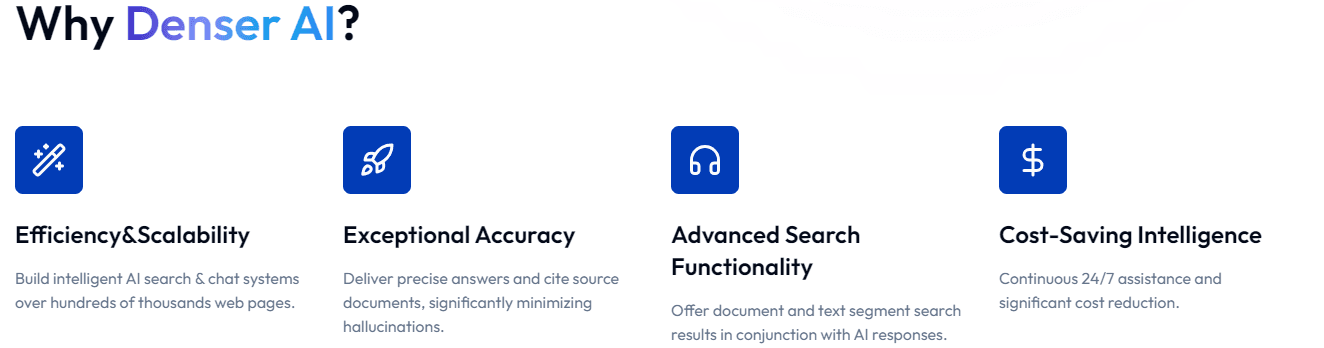
Don’t let valuable data go to waste when it could be helping your business grow. Start using Denser.ai today to train AI models customized to your company.
Try out a free trial or schedule a demo today!
FAQs About Training AI On Company Data
Why are machine learning evaluation metrics important when training AI on company data?
These metrics ensure the model performs well in real-world scenarios. Evaluation metrics help assess how accurately the model is responding to your data. Without using these metrics, you won’t have a clear picture of the model's effectiveness or areas where it needs improvement.
Do I need a large amount of data to train AI?
While having a larger dataset can improve accuracy, you don’t always need massive amounts of data to get started. A well-prepared dataset with a few thousand entries can be sufficient for many use cases.
The quality of your data matters more than the quantity—clean, well-organized, and relevant data leads to better results.
How do large language models differ from traditional machine learning models?
Large language models (LLMs) are generative AI systems that can understand and generate natural language text. Traditional machine learning models, on the other hand, are usually designed for specific tasks like classification or regression.
LLMs are more flexible because they can handle various tasks, from answering customer questions to generating content, especially when trained on company-specific data.
Training a machine learning model on historical customer support tickets can help the AI to quickly recognize common issues and provide instant responses.